As finance departments increasingly integrate AI into their decision-making processes, the importance of understanding AI model reasoning rises exponentially. It’s crucial for finance experts to peel back the layers on inductive, deductive and abductive reasoning in AI to provide the necessary feedback loops for better performance.
Abductive reasoning, in particular, is the essential intersection between the data and human insight. And this intersection is where finance professionals will need to exercise their expertise most.
Induction and Deduction Reasoning in AI Models
Every AI model’s foundation is its capacity to learn from the past and make calculated guesses about the uncertain future. This learning framework is built on the twin pillars of induction and deduction.
Inductive Reasoning
Inductive reasoning is the foundation behind machine learning tools. The model uses inductive reasoning to observe patterns in data and make general rules based on those patterns.
Imagine the AI model as a finance analyst within your company, meticulously combing through years of internal financial reports, hunting for patterns and correlations. By dissecting past business decisions, sales data, market shifts and even employee performance, the model constructs general operational guidelines. For instance, it may identify that certain product lines see reduced sales during particular industry slumps or seasons. This insight becomes a cornerstone of its understanding.
Deductive Reasoning
After recognizing patterns, the AI model then uses deductive reasoning to apply those patterns or general rules to new sets of data.
Post-induction, this is where our AI model steps into the predictive realm. If it detects signs hinting at an upcoming industry slump or changes in consumer preferences, it will infer that those specific product lines may see a dip in sales.
Abductive Reasoning: Where Data Meets Intuition
Beyond induction and deduction, there’s a third player: abductive reasoning. This is where raw data meets intuition and logic. Think of it as the detective work behind the scenes.
Abductive reasoning revolves around formulating the most likely rationale behind a given data point. If an AI system anticipates a sudden surge in a department’s budget, but it doesn’t present an explicit justification, an astute finance analyst—a human—within the company may have further context behind an impending internal initiative, a potential acquisition or a significant project in the pipeline.
Within our intricate corporate environment, abductive reasoning serves as the nexus, fusing the quantitative insights from AI models with the heartbeat of internal dynamics. Human judgment is used to interpret data in light of upcoming product launches, departmental changes, company goals and even organizational strategy shifts.
Scrutinizing AI Predictions with Financial Acumen
The integration of AI in finance doesn’t make the human role redundant; on the contrary, it accentuates the need for skilled professionals. Relying blindly on AI’s word is akin to setting sail without a compass. Abductive reasoning offers the compass, guiding finance professionals through the AI’s predictions.
How can finance teams harness these predictions further?
- Question the AI’s inferences: When the AI model offers an analysis, challenge it. If it’s indicating a dip in sales for a product line, could it be due to a recent internal change? Or maybe there’s a shift in departmental priorities?
- Cross-reference with internal data: Contrast AI’s findings with tangible company metrics, like sales, customer feedback and inventory. If the AI is optimistic about the success of a new initiative, but team feedback suggests challenges in implementation, it’s worth taking a moment to reassess.
- Rely on your knowledge: Trust your instincts. Intuition, molded by years of industry experience, shouldn’t be overlooked. If the AI’s conclusions feel misaligned when compared to historical company trends or your own understanding of the business environment, delve deeper.
- Collaborate with teammates: Discuss the AI’s insights with colleagues and department heads to add depth to the analysis. Group perspectives can shed light on facets of AI analyses that may not be immediately evident to an individual. Maybe the marketing team has a perspective that adds nuance to AI’s sales predictions.
- Iterate and refine: Understand that, despite their advanced capabilities, AI models can be flawed. As business goals shift and market dynamics evolve, continually feed these insights back into the AI models. This recursive loop ensures that the models remain aligned with the company’s evolution. Use insights from abductive reasoning to continually adjust them, ensuring their recommendations grow more accurate with each iteration.
By leveraging abductive reasoning, finance professionals can ensure that they’re not just riding the AI wave but truly steering the ship, ensuring predictions resonate with the dynamics of the business.
About the Author
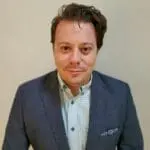
Eli Gill is the Vice President of Engineering, Product, and AI at Paro, an AI-powered marketplace that delivers finance and accounting solutions to businesses through a combination of expert fractional talent, data-driven tools, and guiding insights. Eli has worked in the AI and machine learning field for over 10 years and served for five years as a limited term lecturer in the subjects of machine learning, data science, and AI at Purdue University.